Learning to Solve PDE-constrained Inverse Problems
with Graph Networks
ICML 2022
-
Qingqing Zhao
Stanford University -
David B. Lindell
Stanford University -
Gordon Wetzstein
Stanford University
Video
Abstract
Learned graph neural networks (GNNs) have recently been established as fast and accurate alternatives for principled solvers in simulating the dynamics of physical systems. In many application domains across science and engineering, however, we are not only interested in a forward simulation but also in solving inverse problems with constraints defined by a partial differential equation (PDE). Here we explore GNNs to solve such PDE-constrained inverse problems. Given a sparse set of measurements, we are interested in recovering the initial condition or parameters of the PDE. We demonstrate that GNNs combined with autodecoder-style priors are well-suited for these tasks, achieving more accurate estimates of initial conditions or physical parameters than other learned approaches when applied to the wave equation or Navier-Stokes equations. We also demonstrate computational speedups of up to 90x using GNNs compared to principled solvers.
Overview
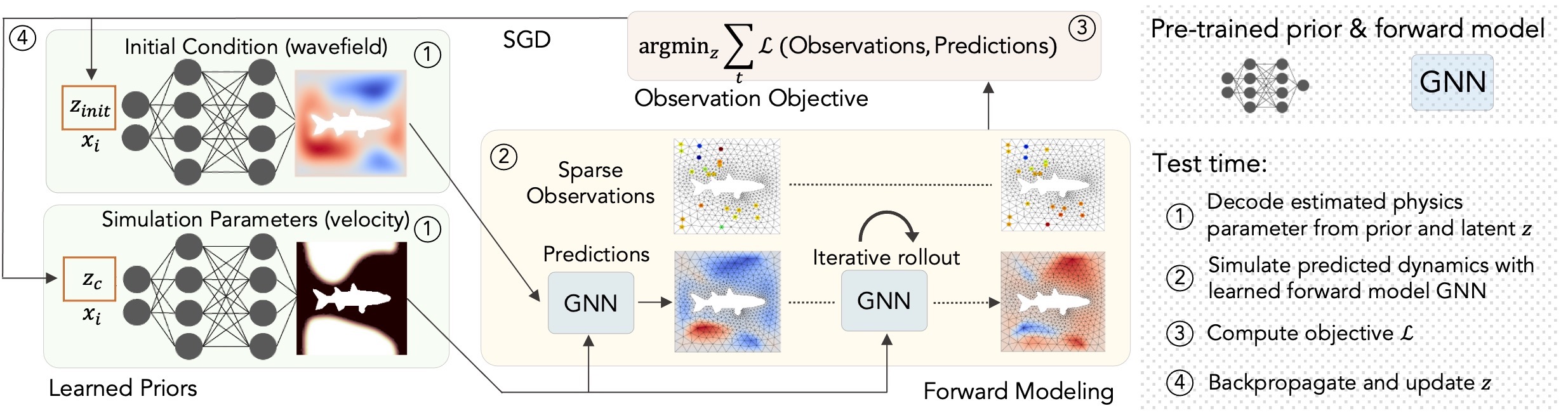
Pipeline for solving inverse problems (illustrated for the 2D wave equation). The forward simulator GNN (the blue boxes) and the prior networks (green boxes) are pre-trained with a dataset of wave trajectories generated using classical FEM solvers. We aim to recover the initial wavefield or the velocity distribution. At test time, the generative model first maps a latent code to the estimated physics parameters (step 1) that is passed to the GNN to obtain the predicted dynamics (step 2). The latent code is optimized to minimize the difference between the predicted and the observed dynamics on the graph (steps 3 and 4).
Download
Citation
@inproceedings{qzhao2022graphpde,
title={Learning to Solve PDE-constrained Inverse Problems with Graph Networks},
author={Qingqing Zhao and David B. Lindell and Gordon Wetzstein}
journal={ICML},
year={2022}
}
Acknowledgements
The website template was borrowed from Michaël Gharbi.